Full Stack AI ML Development Courses
This document provides the curriculum outline of the Knowledge, Skills and Abilities that a Machine Learning Developer and DevOps/MLOps Administrator can be expected to demonstrate.
Prerequisite:
- Python Programming Fundamentals.
- Familiarity with Command Line Interface (CLI) and Git/GitHub, DVC
- Fundamental Knowledge of Django, Database, HTML/CSS.
- Familiarity with at least machine learning data preprocessing, YAML, JSON.
Out Come:
After attending this training, the trainees will be gaining the below skills on Full Stack AI/ML Model Design, Development & DevOps/MLOps Orchestration.
- ML/MLOps Architecture Vs DevOps Framework.
- Install/Configure Cookie Cutter Template and DVC (Data Tracking).
- Build a Custom Environment for ML/MLOps model design and retraining.
- Manage DevOps/MLOps Lifecycle, Storage, and CI/CD Pipeline.
- Installing and Configuring Jenkins and Nagios.
- Installing and Configuring MLFlow, PyTest, and Pep8.
- Deploying and scaling the model Django & Haruko Platform.
- Data Storage using PostgreSQL.
- Auditing and Troubleshooting Machine Learning Model.
- DevOps/MLOPs best Security Practices.
- Complete the Life Cycle of AI/ML Software development using various technologies.
- ML Model in Amazon SageMaker.
- Auto Implement on DataRobot.
Local setup (Physical Mode) |
Remote Lab Setup (Optional) |
|
|
Laptop/Desktop with high-speed |
OS: Windows 10 and Above |
internet connection, Windows 10 and |
|
above |
|
Memory: 4 GB RAM |
Memory: 32 GB RAM |
CPU: 1 CPU Cores |
CPU: 8 CPU Cores |
Storage: 20 GB |
Storage: 500 GB SSD |
Part – 1
Introduction to MLOps:
- What is MLOps?
- Why we need MLOps and business impact?
- Machine learning industrialization challenges
- How does it relate to DevOps, AIOps, ModelOps, and GitOps?
Introduction to ML and MLOps stages:
- What are the various stages in the ML lifecycle?
- Detailed MLOps Principles and stages
- Testing
- Automation (CI/CD)
- Reproducibility
- Deployment
- Monitoring
- Versioning
- MLOps Architectures:
- Architectures \w Open Source tools
- Architectures \w cloud Native tools – Amazon Web Services
- Comparison among cloud native tools
- Cost-benefit approach of each architecture and MLOps maturity
- List of tools involved in each stages (MLOps tool ecosystem)
- MLOps Maturity Model
- Team ownership types in various stages of MLOps
Introduction to Git/Github:
- Overview of Git
- Understanding branching strategies and REPO
- Standard GIT branching strategies (development, feature, bug, release, UAT)
- Practicing important Git commands
- Github Action overview and working
Introduction to CI/CD Using Jenkins:
- Introduction to CI/CD
- CI/CD challenges in Machine Learning
- Steps involved in the CI/CD implementation in ML lifecycle and workflow
- Glimpse of popular Tools used in the DevOps ecosystem on 1 cloud – e.g. AzureDevOps or Cloud Build cloud formationion
Cloud Native CI/CD Tools 101:
- Jenkins on AWS OR Cloud Build or cloud formation
Hands-on:
- Data Set from Kaggle is considered to demonstrate the real time Machine Learning Model Design and Development. (Regression Model)
- ML Model will be retraining with an industry use case showing how CI/CD on an ML Model using DVC.
- Workshop on CloudBuild with an industry use case showing how CI and CD on an ML Model AWS
- Model Testing using Pytest and Tox Dependencies.
Kubernetes/Docker Overview
- Kubernetes overview
- Kubernetes Architecture o Nodes
o Control Plane o API Server
- Kubernetes Resources o Pod
o Deployment o Replica
o Service
o Volumes (PVC)
- Kubernetes Deployment Strategy o Monitoring
o Liveness and Readiness Probes
- Labels and Selectors
- Docker Installation and Deployment.
Hands-on:
- Kubernetes kubectl command practice
- Practice core concepts like Deploying Pod, deployment, ReplicaSet, Namespaces, imperative commands.
- Use YAML construct for declarative commands
- Create and Deploy ML pipeline on Kubernetes
Part – 2
Introduction to Model Management
- What is a Model Management?
- What are the various activities in Model Management?
- High-level overview of below Model Management tools o MLFlow
o DVC
Cloud ML Services
- What is MLFlow
- Various components of MLFlow Services
- Benefits of using MLFlow Services
Hands-on:
- Building ML pipeline in Github Action.
- Deploying model into Github (Action).
- Monitoring Model Performance using Nagios/Heruko/AWS ElasticBeans.
Introduction to Django, PostgreSQL and Model Deployment using Heruko
- Why Django and PostgreSQL is important?
- What are the various types of front end design related to machine learning model?
- Architecture of Django using Python Programming.
- User Data Storage using PostgreSQL Database.
- Model Deployment using Heruko Server
Hands-on:
- Building front end Graphical User Interface using Django, HTML/CSS
- Data Storage using PostgreSQL Database for future retraining of ML Model.
Introduction to Model Monitoring
- Why monitoring is important?
- What are the various types of monitoring related to machine learning model?
- Architecture of monitoring ecosystem in Nagios
- Various monitoring tools on Local Machine/Cloud Platform.
Hands-on:
- Building a drift monitoring system on Nagios.
Introduction to AutoML tools
- H20
- Dataiku
- Domino
- Datarobot
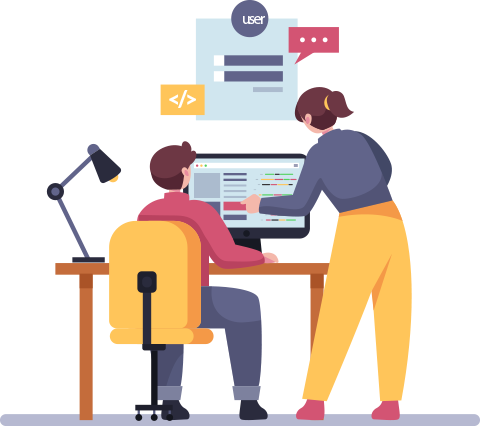